This site uses cookies. By continuing to browse the site, you are agreeing to our use of cookies.
Accept all cookies and servicesDo not acceptLearn moreWe may request cookies to be set on your device. We use cookies to let us know when you visit our websites, how you interact with us, to enrich your user experience, and to customize your relationship with our website.
Click on the different category headings to find out more. You can also change some of your preferences. Note that blocking some types of cookies may impact your experience on our websites and the services we are able to offer.
These cookies are strictly necessary to provide you with services available through our website and to use some of its features.
Because these cookies are strictly necessary to deliver the website, refusing them will have impact how our site functions. You always can block or delete cookies by changing your browser settings and force blocking all cookies on this website. But this will always prompt you to accept/refuse cookies when revisiting our site.
We fully respect if you want to refuse cookies but to avoid asking you again and again kindly allow us to store a cookie for that. You are free to opt out any time or opt in for other cookies to get a better experience. If you refuse cookies we will remove all set cookies in our domain.
We provide you with a list of stored cookies on your computer in our domain so you can check what we stored. Due to security reasons we are not able to show or modify cookies from other domains. You can check these in your browser security settings.
These cookies collect information that is used either in aggregate form to help us understand how our website is being used or how effective our marketing campaigns are, or to help us customize our website and application for you in order to enhance your experience.
If you do not want that we track your visit to our site you can disable tracking in your browser here:
We also use different external services like Google Webfonts, Google Maps, and external Video providers. Since these providers may collect personal data like your IP address we allow you to block them here. Please be aware that this might heavily reduce the functionality and Menus of our site. Changes will take effect once you reload the page.
Google Webfont Settings:
Google Map Settings:
Google reCaptcha Settings:
Vimeo and Youtube video embeds:
The following cookies are also needed - You can choose if you want to allow them:
You can read about our cookies and privacy settings in detail on our Privacy Policy Page.
Legal Information – Impressum
Third ITU/WHO Workshop on “Artificial Intelligence for Health” on Di, 22.01.2019
/in Conferences/by Andreas HolzingerInteractive Machine Learning: Experimental Evidence for the human-in-the-loop
/in Recent Publications/by Andreas HolzingerRecent advances in automatic machine learning (aML) allow solving problems without any human intervention, which is excellent in certain domains, e.g. in autonomous cars, where we want to exclude the human from the loop and want fully automatic learning. However, sometimes a human-in-the-loop can be beneficial – particularly in solving computationally hard problems. We provide new experimental insights [1] on how we can improve computational intelligence by complementing it with human intelligence in an interactive machine learning approach (iML). For this purpose, an Ant Colony Optimization (ACO) framework was used, because this fosters multi-agent approaches with human agents in the loop. We propose unification between the human intelligence and interaction skills and the computational power of an artificial system. The ACO framework is used on a case study solving the Traveling Salesman Problem, because of its many practical implications, e.g. in the medical domain. We used ACO due to the fact that it is one of the best algorithms used in many applied intelligence problems. For the evaluation we used gamification, i.e. we implemented a snake-like game called Traveling Snakesman with the MAX–MIN Ant System (MMAS) in the background. We extended the MMAS–Algorithm in a way, that the human can directly interact and influence the ants. This is done by “traveling” with the snake across the graph. Each time the human travels over an ant, the current pheromone value of the edge is multiplied by 5. This manipulation has an impact on the ant’s behavior (the probability that this edge is taken by the ant increases). The results show that the humans performing one tour through the graphs have a significant impact on the shortest path found by the MMAS. Consequently, our experiment demonstrates that in our case human intelligence can positively influence machine intelligence. To the best of our knowledge this is the first study of this kind and it is a wonderful experimental platform for explainable AI.
[1] Holzinger, A. et al. (2018). Interactive machine learning: experimental evidence for the human in the algorithmic loop. Springer/Nature: Applied Intelligence, doi:10.1007/s10489-018-1361-5.
Read the full article here:
https://link.springer.com/article/10.1007/s10489-018-1361-5
AI, explain yourself !
/in General, Science News/by Andreas Holzinger“It’s time for AI to move out its adolescent, game-playing phase and take seriously the notions of quality and reliability.”
There is an interesting commentary with interviews by Don MONROE in the recent Communications of the ACM, November 2018, Volume 61, Number 11, Pages 11-13, doi: 10.1145/3276742 which emphasizes the importance of explainability and the need for effective human-computer interaction:
Artificial Intelligence (AI) systems are taking over a vast array of tasks that previously depended on human expertise and judgment (only). Often, however, the “reasoning” behind their actions is unclear, and can produce surprising errors or reinforce biased processes. One way to address this issue is to make AI “explainable” to humans—for example, designers who can improve it or let users better know when to trust it. Although the best styles of explanation for different purposes are still being studied, they will profoundly shape how future AI is used.
Some explainable AI, or XAI, has long been familiar, as part of online recommender systems: book purchasers or movie viewers see suggestions for additional selections described as having certain similar attributes, or being chosen by similar users. The stakes are low, however, and occasional misfires are easily ignored, with or without these explanations.
“Considering the internal complexity of modern AI, it may seem unreasonable to hope for a human-scale explanation of its decision-making rationale”.
Read the full article here:
https://cacm.acm.org/magazines/2018/11/232193-ai-explain-yourself/fulltext
What if the AI answers are wrong?
/in General, Science News/by Andreas HolzingerCartoon no. 1838 from the xkcd [1] Web comic by Randall MUNROE [2] describes in a brilliant sarcastic way the state of the art in AI/machine learning today and shows us the current main problem directly. Of course you will always get results from one of your machine learning models. Just fill in your data and you will get results – any results. That’s easy. The main question remains open: “What if the results are wrong?” The central problem is to know at all that my results are wrong and to what degree. Do you know your error? Or do you just believe what you get? This can be ignored in some areas, desired in other areas, but in a safety critical domain, e.g. in the medical area, this is crucial [3]. Here also the interactive machine learning approach can help to compensate or lower the generalization error through human intuition [4].
[1] https://xkcd.com
[2] https://en.wikipedia.org/wiki/Randall_Munroe
[3] Andreas Holzinger, Chris Biemann, Constantinos S. Pattichis & Douglas B. Kell 2017. What do we need to build explainable AI systems for the medical domain? arXiv:1712.09923. online available: https://arxiv.org/abs/1712.09923v1
[4] Andreas Holzinger 2016. Interactive Machine Learning for Health Informatics: When do we need the human-in-the-loop? Brain Informatics, 3, (2), 119-131, doi:10.1007/s40708-016-0042-6. online available, see:
https://human-centered.ai/2018/01/29/iml-human-loop-mentioned-among-10-coolest-applications-machine-learning
There is also a discussion on the image above:
https://www.explainxkcd.com/wiki/index.php/1838:_Machine_Learning
Project Feature Cloud – Pre-Project Meeting and Workshop successful
/in HCI-KDD Events, Projects, Science News/by Andreas HolzingerFrom October, 21-22, 2018, the project partners of the EU RIA 826078 FeatureCloud project (EUR 4,646,000,00) met at the Technische Universität München, Campus Weihenstephan. Starting from January, 1, 2019 the project partners will work jointly for 60 months on awesome topics around federated machine learning and explainability. The project’s ground-breaking novel cloud-AI infrastructure will only exchange learned representations (the feature parameters theta θ, hence the name “feature cloud”) which are anonymous by default. This approach is privacy by design or to be more precise: privacy by architecture. The highly interdisciplinary consortium, ranging from AI and machine learning experts to medical professionals covers all aspects of the value chain: assessment of cyber risks, legal considerations and international policies, development of state-of-the-art federated machine learning technology coupled to blockchaining and encompasing social issues and AI-ethics.
Elsevier Award Artificial Intelligence in Medicine
/in General/by Andreas HolzingerHow different are Cats vs. Cells in Histopathology?
/in Science News/by Andreas HolzingerAn awesome question stated in an article by Michael BEREKET and Thao NGUYEN (Febuary 7, 2018) brings it straight to the point: Deep learning has revolutionized the field of computer vision. So why are pathologists still spending their time looking at cells through microscopes?
The most famous machine learning experiments have been done with recognizing cats (see the video by Peter Norvig) – and the question is relevant, how different are these cats from the cells in histopathology?
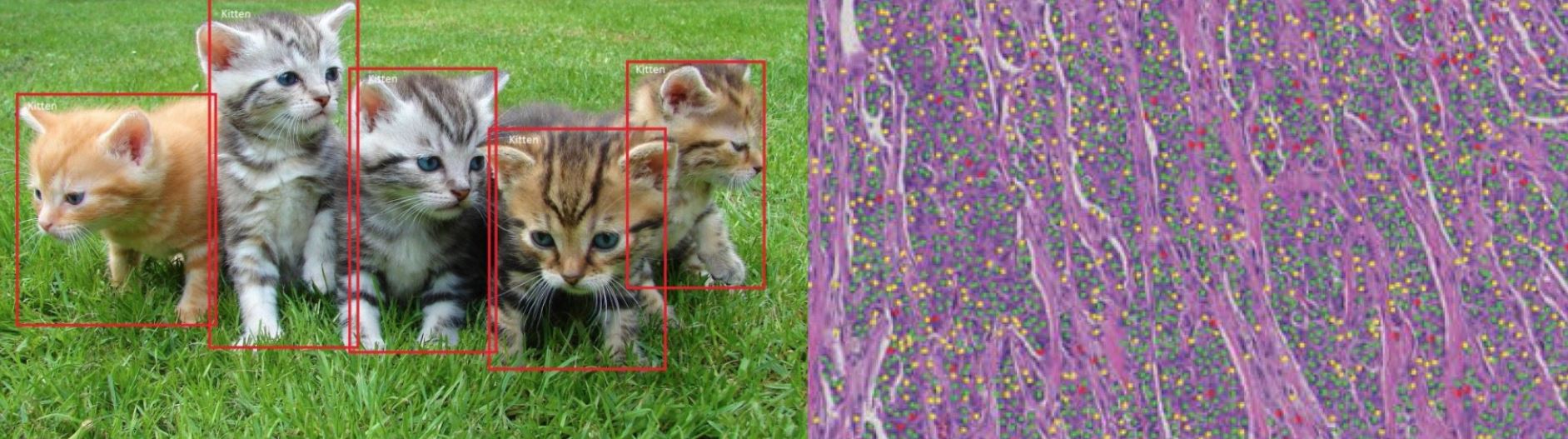
Machine Learning, and in particular deep learning, has reached a human-level in certain tasks, particularly in image classification. Interestingly, in the field of pathology these methods are not so ubiqutiously used currently. A valid question indeed is: Why do human pathologists spend so much time with visual inspection? Of course we restrict this debate on routine tasks!
This excellent article is worthwhile giving a read:
Stanford AI for healthcare: How different are cats from cells
Source of the animated gif above:
https://giphy.com/gifs/microscope-fluorescence-mitosis-2G5llPaffwvio
Yoshua Bengio emphasizes: Deep Learning needs Deep Understanding !
/in Lectures, Science News/by Andreas HolzingerYoshua BENGIO from the Canadian Institute for Advanced Research (CIFAR) emphasized during his workshop talk “towards disentangling underlying explanatory factors” (cool title) at the ICML 2018 in Stockholm, that the key for success in AI/machine learning is to understand the explanatory/causal factors and mechanisms. This means generalizing beyond identical independent data (i.i.d.) – and this is crucial for our domain in medcial AI, because current machine learning theories and models are strongly dependent on this iid assumption, but applications in the real-world (we see this in the medical domain every day!) often require learning and generalizing in areas simply not seen during the training epoch. Humans interestingly are able to protect themselves in such situations, even in situations which they have never seen before. Here a longer talk (1:17:04) at Microsoft Research Redmond on January, 22, 2018 – awesome – enjoy the talk, I recommend it cordially to all of my students!
Explainable AI Session Keynote: Randy GOEBEL
/in Conferences, HCI-KDD Events/by Andreas HolzingerWe just had our keynote by Randy GOEBEL from the Alberta Machine Intelligence Institute (Amii), working on enhnancing understanding and innovation in artificial intelligence:
https://cd-make.net/keynote-speaker-randy-goebel
You can see his slides with friendly permission of Randy here (pdf, 2,680 kB):
https://human-centered.ai/wordpress/wp-content/uploads/2018/08/Goebel.XAI_.CD-MAKE.Aug30.2018.pdf
Here you can read a preprint of our joint paper of our explainable ai session (pdf, 835 kB):
GOEBEL et al (2018) Explainable-AI-the-new-42
Randy Goebel, Ajay Chander, Katharina Holzinger, Freddy Lecue, Zeynep Akata, Simone Stumpf, Peter Kieseberg & Andreas Holzinger. Explainable AI: the new 42? Springer Lecture Notes in Computer Science LNCS 11015, 2018 Cham. Springer, 295-303, doi:10.1007/978-3-319-99740-7_21.
Here is the link to our session homepage:
https://cd-make.net/special-sessions/make-explainable-ai/
amii is part of the Pan-Canadian AI Strategy, and conducts leading-edge research to push the bounds of academic knowledge, and forging business collaborations both locally and internationally to create innovative, adaptive solutions to the toughest problems facing Alberta and the world in Artificial Intelligence/Machine Learning.
Here some snapshots:
R.G. (Randy) Goebel is Professor of Computing Science at the University of Alberta, in Edmonton, Alberta, Canada, and concurrently holds the positions of Associate Vice President Research, and Associate Vice President Academic. He is also co-founder and principle investigator in the Alberta Innovates Centre for Machine Learning. He holds B.Sc., M.Sc. and Ph.D. degrees in computer science from the University of Regina, Alberta, and British Columbia, and has held faculty appointments at the University of Waterloo, University of Tokyo, Multimedia University (Malaysia), Hokkaido University, and has worked at a variety of research institutes around the world, including DFKI (Germany), NICTA (Australia), and NII (Tokyo), was most recently Chief Scientist at Alberta Innovates Technology Futures. His research interests include applications of machine learning to systems biology, visualization, and web mining, as well as work on natural language processing, web semantics, and belief revision. He has experience working on industrial research projects in scheduling, optimization, and natural language technology applications.
Here is Randy’s homepage at the University of Alberta:
https://www.ualberta.ca/science/about-us/contact-us/faculty-directory/randy-goebel
The University of Alberta at Edmonton hosts approximately 39k students from all around the world and is among the five top universities in Canada and togehter with Toronto and Montreal THE center in Artificial Intelligence and Machine Learning.
CD-MAKE Keynote by Klaus-Robert MÜLLER
/in Conferences, HCI-KDD Events, Lectures/by Andreas HolzingerProf. Dr. Klaus-Robert MÜLLER from the TU Berlin was our keynote speaker on Tuesday, August, 28th, 2018 during our CD-MAKE conference at the University of Hamburg, see:
Klaus-Robert emphasized in his talk the “right of explanation” by the new European Union General Data Protection Regulations, but also shows some diffulties, challenges and future research directions in the area what is now called explainable AI. Here you find his presentation slides with friendly permission from Klaus-Robert MÜLLER:
https://human-centered.ai/wordpress/wp-content/uploads/2018/08/cd-make-N-muller18.pdf
(3,52 MB)
Here some snapshots from the keynote:
Thanks to Klaus-Robert for his presentation!